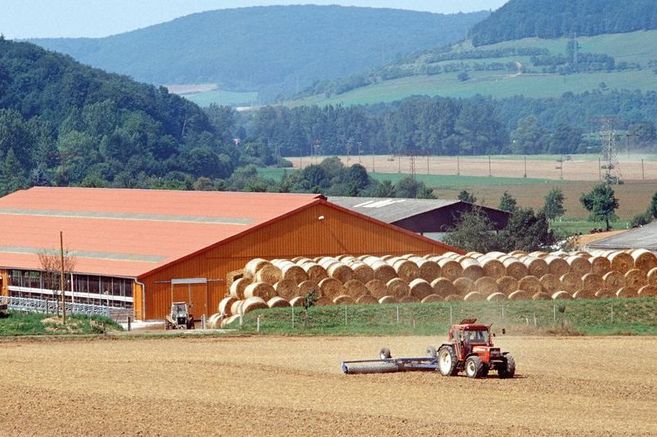

Institut für
BW Betriebswirtschaft
Weitere Publikationen von Marcel Schwieder
- 0
do Nascimento Bendini H, Garcia Fonseca LM, Schwieder M, Sehn Körting T, Rufin P, Del Arco Sanches I, Leitão PJ, Hostert P (2019) Detailed agricultural land classification in the Brazilian cerrado based on phenological information from dense satellite image time series. Int J Appl Earth Observ Geoinf 82:101872, DOI:10.1016/j.jag.2019.05.005
- 1
Leitão PJ, Schwieder M, Pedroni F, Sanchez M, Pinto JRR, Maracahipes L, Bustamante M, Hostert P (2019) Mapping woody plant community turnover with space-borne hyperspectral data - a case study in the Cerrado. Remote Sensing Ecol Conserv 5(1):107-115, DOI:10.1002/rse2.91
- 2
Suess S, van der Linden S, Okujeni A, Griffiths P, Leitão PJ, Schwieder M, Hostert P (2018) Characterizing 32 years of shrub cover dynamics in southern Portugal using annual Landsat composites and machine learning regression modeling. Remote Sens Environ 219:353-364, DOI:10.1016/j.rse.2018.10.004
- 3
Leitão PJ, Schwieder M, Pötzschner F, Pinto JRR, Teixeira AMC, Pedroni F, Sanchez M, Rogass C, van der Linden S, Bustamante M, Hostert P (2018) From sample to pixel: multi-scale remote sensing data for upscaling aboveground carbon data in heterogeneous landscapes. Ecosphere 9(8):e02298, DOI:10.1002/ecs2.2298
- 4
Schwieder M (2018) Landsat derived land surface phenology metrics for the characterization of natural vegetation in the Brazilian savanna. Berlin: Humboldt-Univ, 129 p, Berlin, Humboldt-Univ, Diss, DOI:10.18452/19368
- 5
Schwieder M, Leitão PJ, Pinto JRR, Teixeira AMC, Pedroni F, Sanchez M, Bustamante M, Hostert P (2018) Landsat phenological metrics and their relation to aboveground carbon in the Brazilian Savanna. Carbon Balance Manag 13:7, DOI:10.1186/s13021-018-0097-1
- 6
Leitão PJ, Schwieder M, Senf C (2017) sgdm: An R package for performing sparse generalized dissimilarity modelling with tools for gdm. Int J Geo Inf 6(1):23, DOI:10.3390/ijgi6010023
- 7
Schwieder M, Leitão PJ, da Cunha Bustamante MM, Ferreira LG, Rabe A, Hostert P (2016) Mapping Brazilian savanna vegetation gradients with Landsat time series. Int J Appl Earth Observ Geoinf 52:361-370, DOI:10.1016/j.jag.2016.06.019
- 8
Leitão PJ, Schwieder M, Suess S, Catry I, Milton EJ, Moreira F, Osborne PE, Pinto MJ, van der Linden S, Hostert P (2015) Mapping beta diversity from space: Sparse Generalised Dissimilarity Modelling (SGDM) for analysing high-dimensional data. Methods Ecol Evol 6(7):764-771, DOI:10.1111/2041-210X.12378
- 9
Leitão PJ, Schwieder M, Suess S, Okujeni A, Galvão LS, van der Linden S, Hostert P (2015) Monitoring natural ecosystem and ecological gradients: Perspectives with EnMAP. Remote Sensing 7(10):13098-13119, DOI:10.3390/rs71013098
- 10
van der Linden S, Rabe A, Held M, Jakimow B, Leitão PJ, Okujeni A, Schwieder M, Suess S, Hostert P (2015) The EnMAP-Box - A toolbox and application programming interface for EnMAP data processing. Remote Sensing 7(9):11249-11266, DOI:10.3390/rs70911249
- 11
Suess S, van der Linden S, Okujeni A, Leitão PJ, Schwieder M, Hostert P (2015) Using class probabilities to map gradual transitions in shrub vegetation from simulated EnMAP data. Remote Sensing 7(8):10668-10688, DOI:10.3390/rs70810668
- 12
Schwieder M, Leitão PJ, Suess S, Senf C, Hostert P (2014) Estimating fractional shrub cover using simulated EnMAP data: A comparison of three machine learning regression techniques. Remote Sensing 6(4):3427-3445, DOI:10.3390/rs6043427
- 13
Buchhorn M, Walker DA, Heim B, Raynolds MK, Epstein HE, Schwieder M (2013) Ground-based hyperspectral characterization of Alaska tundra vegetation along environmental gradients. Remote Sensing 5(8):3971-4005, DOI:10.3390/rs5083971